I read Rishi Narang’s Inside the Black Box as a kind of primer on quantitative trading, in advance of reading Michael Lewis’ Flash Boys.
Need for a primer on quantitative trading
I need a primer before reading Flash Boys for several reasons.
I was an ‘over-the-counter’ institutional bond guy, rather than a participant in the exchange-traded stock markets that form the core of many quantitative trading strategies. In addition, my Wall Street experience is a decade old at this point, (I left Goldman in 2004) and the development of dark pool trading, high-frequency trading (HFT), and alternate electronic exchange trading has proliferated since then.
Whereas the traditional stock exchanges with real-live human specialists (like the New York Stock Exchange – NYSE) was already in decline by the time I left Wall Street, the vast majority of stock-trading volumes now happen electronically on alternate exchanges and dark pools away from the traditional NYSE.
As I noted a few years ago, most stock-trading volume is entirely devoid of human decision-making, which is to say, computer-programmed algorithms determine what trades happen on a second-by-second, moment-by moment basis.
Finally, I have a few friends in the quantitative trading world and one of them reached out to me shortly after the publication of Lewis’ book and said there are laughably wrong statements and implications in Flash Boys. In the interest of having an intelligent dialogue with him, to figure out if Lewis really screwed up, I needed some background.
Different Audience for the Books
Narang’s audience for his book differs from Michael Lewis’ audience for Flash Boys. We rely on Lewis for funny anecdotes and characters, and enough financial sense to give the general public an accurate, entertaining, and non-technical narrative.
By contrast Narang’s book targets non-expert practitioners who need more quantitative trading knowledge such as: new hires into the quantitative trading world – or current and prospective investors/asset allocators to quant-trading funds. Narang currently is an investor/allocator to quantitative strategies, who previously founded, and ran, a quantitative trading firm directly.
Narang provides a simplified ‘how to understand’ or ‘how to evaluate’ quantitative trading firms, for readers without specialized trading and computer programming background.
When I say ‘simplified’ I don’t mean the book will be ‘simple’ for people outside finance. Narang’s language is clear and precise, even full of useful analogies for explaining concepts, but it’s academic in style. He systematically reviews, engineer-like, the common components and varieties of quantitative strategies.
I learned a ton, but folks without a need to know about quant trading will not rip through this like they would a Michael Lewis book on finance.
I had a number of questions about quant trading before I read Inside the Black Box and Narang sheds light on my questions.
What is quantitative trading and what should we know about it?
Quant trading, in its simplest definition, can be distinguished from discretionary trading by the role of human decision-making. In traditional, discretionary, investing we decide to sell or buy a financial instrument for any number of good or bad reasons. With quant trading, by contrast all of the human inputs occur ahead of time, that is to say, through the programming of a trading algorithm to look for and respond to the optimal opportunities to trade. The actual order to sell or buy occurs in response to the signals, rather than any in-the-moment human decision.
How big is quant trading compared to discretionary trading in the markets?
Narang cites a study from 2009 that indicated 60% of trading volume in US equities came from quantitative trading, as well as 45% of trading volume in European exchanges. So if 6 billion US shares changed hands per day, then Hal9000 algorithm-based trades accounted for 3.6 Billion of those, leaving only 2.4 Billion shares traded by human discretion. In addition, over 90% of commodity trading firms (CTAs) are quant-trading firms, rather than discretionary traders.[1]
So how do programmers and traders set up the trading algorithm to order trades ahead of time?
Naturally, the next question is about how this programming gets done, and answering this question forms the bulk of Inside the Black Box.
Narang comprehensively reviews and explains both the common elements of building a quant trading strategy, as well as the wide variety of approaches used by the entire spectrum of quant trading firms.
Typically, programming a successful trading algorithm depends on reviewing extraordinary amounts of historical data on observed trading responses of financial instruments, and then seeking patterns among the noise. If a quant trader can find predictable enough responses, then it becomes possible to test if trading on an expectation that the pattern continues could be profitable over time. If his algorithm recognizes the pattern well enough, he can, in effect, ‘see the future’ enough to trade predictably and profitably.
So, it’s pattern-seeking?
A few simple examples of patterns may be helpful here to give an introductory idea. Some stocks – often from the same industry – predictably track each other’s direction, such that a directional move from one typically will be mirrored by a directional move in the other. A similar pattern could hold for a single stock and a ‘basket’ of similar enough stocks.
Volume data on stocks – how much is being traded over any given time period – may predictably be followed by moves in the stock or financial instrument.
Some directional trends in financial instruments could indicate a predictable pattern of a continuation of that trend, or just as likely (and somewhat paradoxically) the likely reversal of a trend.
The common denominator in this type of trading, however, posit that past trading data indicates somehow what will happen in the near future.
To the extent that markets behave in patterned ways – and in fact most of the time financial markets do behave in recognizable historical patterns, or at least recognizable to quants and their computers – quant traders can make money with trading algorithms. This doesn’t have to happen every time, with every trade or every day. In fact, quant strategies depend not so much on winning on every trade, but winning on enough trades to overcome the losses on other trades, as well as the costs, or ‘trading friction,’ of executing many trades simultaneously.
Some of the time, of course, market regimes may shift and past patterns become poor indicators of future moves. At that point, the human-element decision becomes whether to lower the trading exposure based on the algorithm, to shut down the algorithm altogether, or to reprogram the algorithm in response to updated market conditions and patterns.
My sense from reading Narang’s book, as well as from talking to friends in the business, is that quant traders must engage in constant vigilance around their algorithms: Algorithms may cease to generate positive returns as competition eliminates the opportunity, or competitors’ speed eliminates the previous advantage, or patterns simply stop working.
Research and development to find new ‘signals’ inside the noise of past trading data requires constant work.
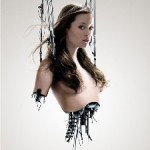
Besides pattern-seeking and constant R & D, what are other elements common to the wide variety of quant traders?
DATA – The acquisition of historical data, the scrubbing of data to extract useful information, and the testing of hypotheses (will following that pattern actually make money?) using the historical data are common to all quants.
COSTS – Some strategies require thousands or even millions of trades, so costs associated with trading matter a lot. Quants have to include in their model expected trading costs – not only commissions paid to brokers or exchange – but the ‘slippage’ that occurs due to trading. The scientific method analogy to this ‘slippage’ here would be the “Hawthorne Effect” or “Observer Effect,” meaning it’s difficult-to-impossible to collect ‘profit’ from the market without affecting the market opportunity itself.
When a quant finds a financial instrument momentarily ‘cheap’ according to some historical pattern, the act of purchasing the instrument to take advantage of the cheapness may cause the price to rise.
The larger the trade that the quant algorithm demands to take advantage of that momentary cheapness, the larger the ‘slippage’ due to the trade itself will actually eliminate the opportunity. Any historical model that fails to take into account this cost element will likely fail to work.
SCIENTIFIC METHOD – Quant-trading, more than discretionary trading, depends on a ‘scientific method’ approach to investing. While a discretionary trader may depend on fuzzy thinking and ‘gut feeling’ to guide his buy and sell signals, quant trading eliminates this part of trading in favor of a rigorous Hypothesis à Test à Hypothesis à Test continuous feedback loop, with consistent profitability (in trading-speak, the generation of “Alpha,” or excess return over risk) the primary indicator of whether the hypothesis has any validity in real markets. Like scientific hypotheses, the trading algorithm can never become ‘proven’ or ‘permanent law.’ In fact, many current predictors of market moves may be shown to be poor predictors in the future when markets change. A continuous testing of hypotheses about patterns through the scientific method helps distinguish quants from discretionary traders.
How different is High Frequency Trading (HFT) from other types of quant trading, and what should we know about the subset of quant trading known as HFT?
Narang devotes the final chapters of this book to explaining HFT. Narang suggests that the trading ideas of HFTs can often be much simpler than other quant trading – but depend on faster execution of the simpler idea.
Narang provides a relatively easy illustration of a typical, simple, HFT idea.
The S&P500 index consists of shares from the 500 largest market-capitalization US companies, in known relative weightings. Naturally whenever the price of an individual stock in the index changes, the value of the S&P 500 index as a whole changes slightly as well. An exchange traded fund (ETF) like the SPY, or an S&P500 futures contract (ES), both of which are meant to track the index precisely, should exactly mirror the price changes in the underlying stocks.
If, however, one of the underlying stocks goes up in value and the SPY value does not change precisely, instantaneously, to a new value based on the moves in the underlying stocks, a super-fast arbitrage can be had by buying the SPY and shorting the stock. These arbitrage opportunities are either rare or exist for very brief instances – all sorts of traders would look for precisely this simple risk-less opportunity – but trading speed makes exploiting a price difference like this possible.
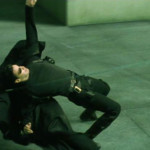
The best way to explain this is that only a computer programmed to move as fast as Neo in that scene dodging Mr. Smith’s bullets, could hope to profit from the S&P/ SPY arbitrages. Nobody else has a chance.
The basic trading idea here is super-simple. On the other hand the technology involved in calculating the precise price discrepancies, in the right weightings, based on the right data feeds, and doing it faster than anyone else in the marketplace, is not easy.
The S&P 500 trade described above is probably version 1.0 of HFT trading, and most HFT firms seek out similar price opportunities with fewer competitors and less efficient instruments in myriad markets around the world.
HFTs may seek to use more complicated algorithms than this example, but at a certain point the computing time required to sift through the market signal may slow down the process, so that they begin to resemble other quant traders.
By keeping the trading idea dumb and simple, the HFTs retain the advantage that comes from technology. HFT is the Keanu Reeves of quant trading, dumb and dependent on Speed to keep the bus above 88 mph to prevent everything from exploding.
Conventional quants may have harder-to-explain patterns that they recognize and exploit, but probably do not depend on execution speed to the same extent as HFTs in order to be profitable.[2]
How likely, and with what severity, could we experience a destructive flash-crash catastrophe driven by the present and future take-over of markets by quant trading? In other words, should we the public (and regulators) be much more actively regulating SkyNet?
Narang addresses a version of this question in Chapter 10, “Risks Inherent to Quant Strategies,” although his goal is limited to showing – persuasively in my opinion – that large market blow-ups in the past cannot be blamed on quants.
The Long Term Capital Management (LTCM) blowup of September 1998, for example, may be described by some as a quant-fund disaster that fundamentally shook capital markets, and might have gotten worse without a Federal Reserve-Inspired ‘Bail-in’ of LTCM by Wall Street firms.
Narang points out, however, that while LTCM used sophisticated quantitative models in their trading, their strategy remained fundamentally discretionary – meaning humans made the calls on what went into and out of their portfolio.
The 2008 Mortgage-bond inspired crisis, in addition, cannot reasonably be linked to quant trading firms. While the creation and trading of highly-leveraged mortgage and default-swap CDOs involves sophisticated mathematics and modeling, the billions in losses owed more to a combination of leverage (debt), human error, poor risk-modeling, the connectivity of markets and counter-parts, and misunderstood incentives among disparate parts of the mortgage-bond origination chain than anything to do with quantitative trading – as explained well in Michael Lewis’ The Big Short.
Arguably, the most significant systemic market risk crash to date that could be blamed on quant-like trading is the October 1987 market crash, in which computer-based “portfolio-insurance” algorithms each triggered a cascade of automatic selling, independent of human judgment. The Crash of 1987 occurred prior to the rise of quant trading as we know it, so ‘blaming’ quant trading can really be done only by historic analogy. It’s the kind of crash we could imagine from algorithmic trading in which the size of computer trading orders, added to the overwhelming one-sidedness of the computer signal (a crowded trade) causes the devastation.
The actual quant trading blowups to date, as Narang explains, have caused localized losses without inflicting much observable or systemic market disruption.
During a few weeks in August 2007, for example, a majority of algorithmic traders simultaneously experienced sudden losses, as the trends suggested by historic patterns of trading relationships reversed. It turns out that the similarities in quantitative strategies at that time meant that as some quants lost money and unwound their portfolios they caused a cascade of losses in other quants’ positions.
In the meantime, the non-quantitative investment community – people who just own stocks or mutual funds, or non-quant hedge funds – barely noticed any particular change in market prices.
The market as a whole neither soared nor crashed. Only the historic patterns of short-term trading relationships broke down and then suddenly and severely reversed. As a silent market-crisis, only experienced by the quant community, August 2007 may be a harbinger of the kind of localized pain that non-quants can safely ignore. Or conversely, the August 2007 crisis indicates the growing power and ‘crowded trade’ nature of quant trading that regulators should worry about which could freeze up or destroy orderly markets in the future.
Other flash crashes, such as the May 2010 Flash Crash or other mini-crises such as happened in August 2012 that brought down Knight Trading, may fit the pattern of acceptable, localized, market blips. Optimistically, programming errors like those are just a Darwinian way of punishing the inept and incentivizing the careful and level-headed to take advantage of others’ mistakes.
Or, they could be the early tremors in market-structure fault-lines created by quant trading that will eventually cause an irreversible earthquake in world markets. I don’t want to be alarmist on a topic where I’m an amateur, but I hope some regulators have taken on the ‘seismic geologist’ role of figuring out how to predict and prevent ‘The Big One’ caused by quant trading, before it happens.
I mean, we’ve all seen 2001 Space Odyssey, Tron, Terminator, and The Matrix, right, so we know this doesn’t bode well for algorithmic trading. Even Aliens and Planet of the Apes are basically warnings about quant trading, aren’t they? Wait, am I overthinking this?
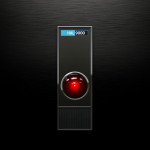
Narang does not particularly address this much more troubling, forward-looking question, of whether and to what extent quants will significantly blow up markets in the future. His primary response in Chapter 16 to this worry or criticism points out that human errors have more frequently caused losses or mini-crashes in the markets than computer glitches. No doubt this is true, but that’s a bit like saying we shouldn’t worry about tornadoes because hurricanes have caused more damage up until now.
As we learned from the 2008 Crisis, markets have a way to experiencing the near-statistically impossible, stunningly unlikely, “6-sigma” event, or “1,000-year flood” just about every decade or two, causing massive wealth destruction in new and unpredictable ways.
Often we can see, in retrospect, that the root cause of a market crash has something to do with the rapid advance of a profitable financial technology far ahead of our ability to control all the risks of the financial technology or regulate the inequities or societal costs of the financial technology.
I’m not in a great position to evaluate the probability and consequences of a future algorithmic-trading driven market catastrophe, and perhaps Narang is not either, although he’s certainly better positioned than most.
I do remain worried about a future SkyNet-driven Crash.
Is quantitative trading in general, or HFT in particular (as the rapidest version of quantitative trading is known) inherently unfair, unethical, or taking advantage of other market participants?
I have not read Flash Boys yet, but I know enough about the plot line to see Michael Lewis’ book as a call to action about the inequities inherent in HFT, a subset of quantitative trading. Whether he’s right or not, regulators are already responding to their perception that HFTs are gaming the system in a way that’s harmful to markets overall.
Narang clearly feels that quantitative trading is an interesting and honorable way to make a living. In the final section of the book, he describes the methods and implications of High Frequency Trading, with noted contributions from his brother Manoj Narang, the CEO of a HFT firm Tradeworx and sometime commenter on the industry.
Given Narang’s experience and connection with the industry, we would not expect him to point a finger at quants or HFTs and declare them inherently unfair. What he does try to do instead is educate the non-expert in how quants may make money, and why HFTs in particular feel the need for speed.
To give one example of this need for speed, his brother’s firm Tradeworx researched the profit difference between placing purchase or sale orders first, versus placing them later in the queue for order filling. Inherent to equity trading, he explains, is the rule that when buy or sell orders get placed on an exchange at the same price, the first order placed gets filled first. The value of being first with certain types of orders, according to Tradeworx, turns out to be approximately 1.7 cents per share.
With other kinds of orders, they have found that immediate, speedy, fulfillment of the trade reduces the cost or ‘slippage’ due to trading. In a strategy dependent on a massive amount of trading to capture momentarily fleeting price reactions, the high speed fulfillment of orders matters tremendously.
How do HFTs get so fast?
In the same chapter, Narang mentions the advantage of locating HFT trading servers at, or near, market exchange servers, a major theme of Flash Boys.
Along a similar vein, Narang reports the lengths and costs endured by trading firms to reduce data transmission times between major trading centers such as New York, Chicago, and London. HFT trading firms will sign long-term, multi-million dollar contracts for fiber optic access in order to shave off five milliseconds (there are 1,000 milliseconds in every second, so five milliseconds is 5 thousandths of a second!) in data transmission times between New York and London, as an indication of the importance of being the first to post, or first to cancel, transaction orders.[3]
HFT firms, Narang points out, monitor data and order trades in tenths of a millisecond, creating myriad technical challenges, between hardware, software and network engineering.
Another speed bump in the way of the highest frequency trading, Narang notes, has been the regulatory rule adopted in July 2011 that US equity brokers must enact a ‘risk check’ for every trade, in order to ensure that the size and capacity of the customer and the trade are valid – meaning not too big, which might indicate a trade made in error.
The brokerage software required to do this risk check slows down every trade, which could dampen HFTs speed by 0.5 milliseconds. In response, some HFT created their own brokerages, in order to reduce the time-lag of using someone else’s risk check system.
Where do I differ from Narang’s perspective?
1. This is a bit nitpicky, but I have a philosophical issue with Narang’s definition of ‘value’ and the role of ‘timing’ in investing. He argues in both Chapter 3 and Chapter 16 that all investors, all the way from HFTs on the one side of the spectrum to long-term investors such as Warren Buffett on the other side of the spectrum, depend on timing to beat the market. He means that investors have to execute their purchase (or sale) in advance of others’ execution, and it’s this earlier-than-others’ action that creates value.
In Narang’s presentation, the good investor buys first, others follow suit later, and the original investor can then sell at a higher price based on the fact that others have driven up the price of the instrument.
Here I think Narang’s missed the point of value-investing as advocated by Buffett: Value is created by purchasing future cash-flows at an attractive price, and value investors are mostly indifferent to other investors’ subsequent actions. If you conceive of stock ownership with a perpetual time horizon, as a value investor like Buffett seems to, and that value derives from those future cash flows purchased at a good price, then timing falls out nearly completely as a consideration.
With a quant’s focus on short-term price changes rather than the cash-flow potential of financial instruments, I can see why Narang views timing as a key to all investing, but I think that focus leads to inaccurately describing other types of long-term investing.
2. I cannot tell from what I read and really this book doesn’t say – whether a constant stream of high frequency orders and cancelled orders constitutes a confusing ‘spamming’ of equity market exchanges, to the detriment of slower investors. Rapid fire orders, the non-quant critics say, create the illusion of market liquidity where it doesn’t really exist, as it may disappear quickly when non-quants actually seek to trade.
I can understand the way in which HFTs seeking arbitrage opportunities between linked instruments (like the S&P500 and the SPY) create liquidity and efficiency to some extent, in a way that may benefit the smooth running of markets.
In addition, Narang claims that rapid order cancellation is a necessary component of HFT strategies[4] and he claims that no evidence supports the complaints from non-quants about damage done by this type of activity.
But do millisecond orders and cancelled orders jam up the orderly execution of others? Does algorithmic swarming of orders around non-quant investment flow hurt the non-quants in a fundamentally unhelpful or unfair way?
Clearly some non-quants traders and investors believe they are harmed by excessive order-making and order-cancellation, when it comes to trading. Are the non-quants being overly paranoid or jealous of HFT profits, or is there actual harm done? Basically I need a non-quant trader’s opinion here, and I don’t think Narang sufficiently addresses this type of externality of HFT activity.
3. I’ve already mentioned that his book does not dwell on the potential for future catastrophic losses, nor did I expect it to, as the future is unpredictable and Narang would be engaging in speculation about the unknown. On the other hand, we’ve had enough mini-shocks in the market attributable to algorithmic trading that I hope someone, somewhere (ideally in a government regulatory office) is thinking deeply about ways to prevent The Big One from happening.
Please see related book review: Flash Boys, by Michael Lewis
as well as Flash Boys – Not So Fast by Pete Kovac
Please see related post: The Rise of The Machines
[1] People, what I’m trying to say is: SkyNet already took over the commodities and equity markets. Good thing there’s some real live ex-bankers who still write finance blogs. Ha ha. Or maybe that’s just what I want you to think.
[2] Maybe in this analogy traditional quant trading is a more complex George Clooney-character? I don’t know, still working on it.
[3] I don’t generally begrudge hedge fund traders anything, but now I’m starting to think they never have to wait those annoying 10 seconds for Game of Thrones to load up on Amazon Prime, like I typically do. Damn, I hate those guys. If I was a well-compensated hedge fund trader right now I would happily pay 12 million dollars just to eliminate that delay.
[4] to avoid a problem he explains well, which is the ‘negative selection’ of passive orders on an exchange.
Post read (12853) times.