One of the things that makes finance endlessly fascinating (to me!) is that perfectly sound logic for one situation turns out to be perfect madness in another situation.
In my best moments I appreciate the ironies and contradictions. In my worst moments I despair for people whipsawed by the seeming complexities of financial choices.
Most middle-class folks grapple with one of these important choices – a home mortgage – at least once in their life. I’m a big fan of the choice to buy a home with a mortgage but even there, a controversial battle rages.
Anti-debt
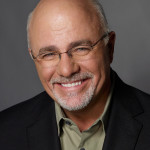
On one side of the ring stand the anti-debt gurus like Dave Ramsey. While Ramsey really wants his followers to pay cash for their homes (which is fairly absurd), he has strong rules about what to do if you decide to borrow. For example, Ramsey says
- Always make at least a 20% down payment, to avoid high interest charges and expensive private mortgage insurance.
- Always get a 15-year mortgage rather than a 30-year mortgage because you will pay it off sooner, typically enjoy a lower interest rate, and you’ll pay significantly less interest over the life of the loan.
- Never take on mortgage debt with a monthly payment that will command more than 25% of your take-home pay.
- Always avoid adjustable rate mortgages which shift the risk of higher interest rates from the lender to you.
- Never borrow additional home equity in the form of a home equity loan or line of credit.1
Ramsey – who built a real estate fortune and then went bankrupt by the age of 30 – preaches a low-debt or (preferably) no-debt financial lifestyle as a curative for people with past debt problems. He knows of what he speaks, and he has a certain strong logic for his points.
On the other hand, personally, I’ve broken each and every one of his rules. So I can’t actually advocate following his advice.
Pro-debt
On the opposite side of the ring, another financial guru Ric Edelman advocates the opposite approach.
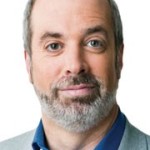
Since Edelman’s contrarian position flies in the face of conventional wisdom, I enjoy presenting his points even more.
- Only make the bare minimum down payment on your house – thereby freeing up your remaining capital for investing in the market, where you can earn an annual return higher than what you pay on your mortgage debt.
- Always get a 30-year mortgage rather than a 15-year mortgage, to take advantage of the tax deduction on mortgage interest.
- Never pay off your mortgage early or at all, because mortgages are the best way to borrow extremely cheaply. Again, use the borrowed money to invest profitably in the market.
- If the value of your house rises, consider freeing up the equity to invest, through a home equity loan or line, rather then let your net worth stay locked up and unused in the form of your house. That way, Edelman says, if the value of your house drops you’ll at least have withdrawn the money and have use of it for emergencies.
- Quickly paying down and eliminating your monthly mortgage payment is not an important goal because, as a homeowner, you’ll always have to pay insurance and real estate taxes anyway. Since you can’t eliminate those obligations, why bother trying to eliminate your mortgage payment?
You get the idea. When Ramsey says “Zig” Edelman says “Zag.”
Edelman presents some compelling math for his arguments. If you accept his assumptions then you could end up wealthier in the long run.
However, Edelman does not account for the psychological difficulty of saving money. Specifically, many of us benefit from the ‘forced savings’ of paying a mortgage, and few will have the discipline to take the extra monthly cash flow as a result of a 30 year mortgage and invest it for the long run, rather than squander it on iced latte frappuccinos.
As a result, I’m pretty sure some portion of people who take Edelman’s advice to heart will end up like the proverbial broke guy having to wear a barrel for pants. It kind of all depends on your specific situation.
My choices
In my own life I’ve had both adjustable rate mortgages and fixed rate mortgages. I’ve borrowed more than the conventional 80% limit. I’ve had 15-year and 30-year loans. I’ve paid extra principal on a biweekly basis, and I’ve also borrowed heavily against my home equity line of credit. I’d like to think I had compelling logic for each decision, or at least a sober mind for understanding what I was doing.
How to decide
I think my point is that the more wholly convinced a guru is, the less certain you should be. The stronger they lean in, the less likely they are to be correct in all circumstances, for all people. Ramsey’s got a great plan, for example, for people who’ve been bankrupt in the past or who have a history of debt problems. Edelman’s approach is closer to my own experience because he’s linking some risk-taking to long-term wealth creation, which I tend to do in my own life. But where you fall on the risk spectrum is a key determinant of their relevancy to your own situation.
Big Ideas vs Little Ideas
Nate Silver’s 2012 book The Signal And The Noise presents the dichotomy of a guru or pundit’s ‘big idea’ vs. ‘small ideas.’ While punditry rewards people who have ‘big ideas’ and ‘hot takes’ on topics, the reality is that certainty and big ideas come at a cost. Predicting the future – one of Nate Silver’s specialties – is a difficult business for people with big ideas. They rarely get it right. Instead, Silver advocates adopting a nimbler approach to observing the world.
When I read gurus like Ramsey and Edelman, I remind myself that their certainty is a sign of the ‘big idea’ thinking that Silver warns against, when we might be served better by smaller ideas, more responsive to changing conditions.
The more certain I am, the less likely I am to be wholly right.
A version of this post ran in the San Antonio Express News.
Please see related posts:
Book Review: The Signal And The Noise by Nate Silver
My 15-year mortgage – I am a Golden God
Home Equity Lines of Credit are awesome
The Latte Effect in my own life
Post read (3725) times.
- I have strong pro-HELOC views, as I’ve written about in the past. ↩